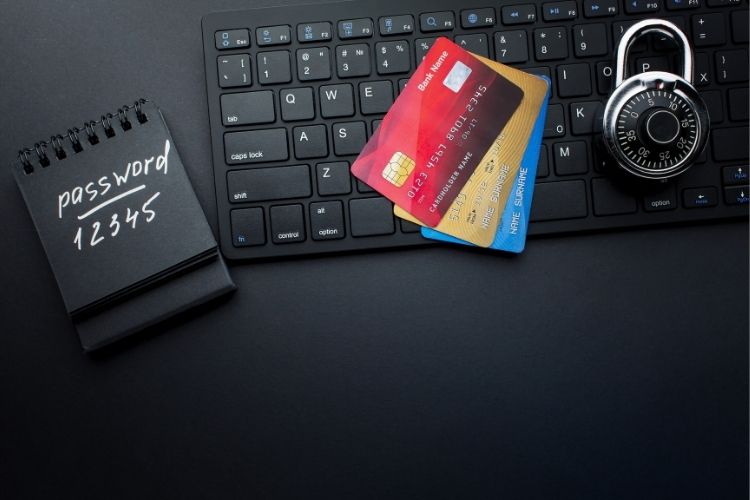
The Rights of the Deceased: Moral Rights Incidental to Copyright Law
- Vanshika Agrawal
- 2024-04-25
The advent of artificial intelligence (AI) in credit assessment has transformed the banking landscape, offering efficiency and precision. However, concerns about bias and fairness in AI algorithms have become significant considerations for regulators, especially in the Indian banking sector. This article explores the current scenario of AI in credit assessment within Indian banking regulations, focusing on the challenges of bias and the regulatory measures in place to address them.
The Rise of AI in Credit Assessment:
AI-powered algorithms have become integral in automating credit assessment processes. These systems analyze vast amounts of data to evaluate an individual's creditworthiness, providing quicker decisions and expanding financial inclusion.
Challenges of Bias in AI Algorithms:
Despite the benefits, AI algorithms are susceptible to biases present in historical data. If the training data used to develop these algorithms contains biases, the AI system may perpetuate and amplify those biases, leading to discriminatory outcomes.
The Reserve Bank of India (RBI) has recognized the potential of AI in enhancing efficiency and customer experience in the banking sector. The RBI's regulatory framework encourages the adoption of AI while emphasizing the need for responsible and fair practices.
Regulators [1]in India acknowledge the challenges associated with bias in AI algorithms. The diversity of the Indian population poses a unique set of challenges, requiring regulators to ensure that AI systems are fair and unbiased across various demographic groups.
The RBI has issued guidelines emphasizing fair practices in lending, including the use of AI in credit assessment. These guidelines stress the importance of transparency, customer education, and the need to ensure that AI systems do not result in discriminatory practices.
The Personal Data Protection Bill, currently under consideration, aims to establish comprehensive data protection laws in India. These regulations will play a crucial role in governing the use of personal data in AI algorithms and mitigating privacy concerns.
To mitigate bias, AI algorithms should be trained on diverse and representative datasets that encompass the demographics of the Indian population. Ensuring that the training data reflects the country's diversity helps reduce the risk of biased outcomes.
Regulators emphasize the importance of explainability and transparency in AI algorithms. Banks are encouraged to adopt models that provide clear insights into the factors influencing credit decisions, allowing regulators and customers to understand the process and identify potential biases.
Continuous monitoring and auditing of AI systems are essential to identify and rectify biases that may emerge over time. Regulators may require banks to implement mechanisms for ongoing assessment and correction of bias in their AI algorithms.
The incorporation of ethical considerations in AI development is crucial. Regulators can encourage banks to adopt ethical AI practices, including the establishment of ethical AI committees, adherence to fairness standards, and regular ethical reviews of AI models.
Defining fairness in credit assessment becomes complex in a diverse country like India. Regulators need to navigate cultural, social, and economic nuances to develop a comprehensive understanding of fairness that is applicable across diverse segments.
The rapid evolution of AI technology requires regulators to be agile and responsive. Regular updates and revisions to regulatory frameworks may be necessary to address emerging challenges and advancements in AI systems.
Enhancing consumer awareness about AI in credit assessment and educating them about their rights and the functioning of these algorithms is essential. Regulators can collaborate with banks to implement initiatives that promote consumer education on AI-related processes.
As AI continues to shape credit assessment in the Indian banking sector, regulatory frameworks must strike a balance between fostering innovation and ensuring fairness. Addressing bias in AI algorithms requires a multi-faceted approach involving diverse and representative data, transparent practices, and continuous monitoring. The RBI's guidelines and potential data protection regulations signal a commitment to creating an environment where AI contributes to financial inclusion while upholding ethical and fair practices in credit assessment. The evolving landscape of AI in Indian banking underscores the need for ongoing collaboration between regulators, banks, and the broader community to build a trustworthy and unbiased AI-powered financial ecosystem.
[1] https://www.niti.gov.in/sites/default/files/2021-02/Responsible-AI-22022021 (last visited Jan. 21, 2024).
Drop your comment